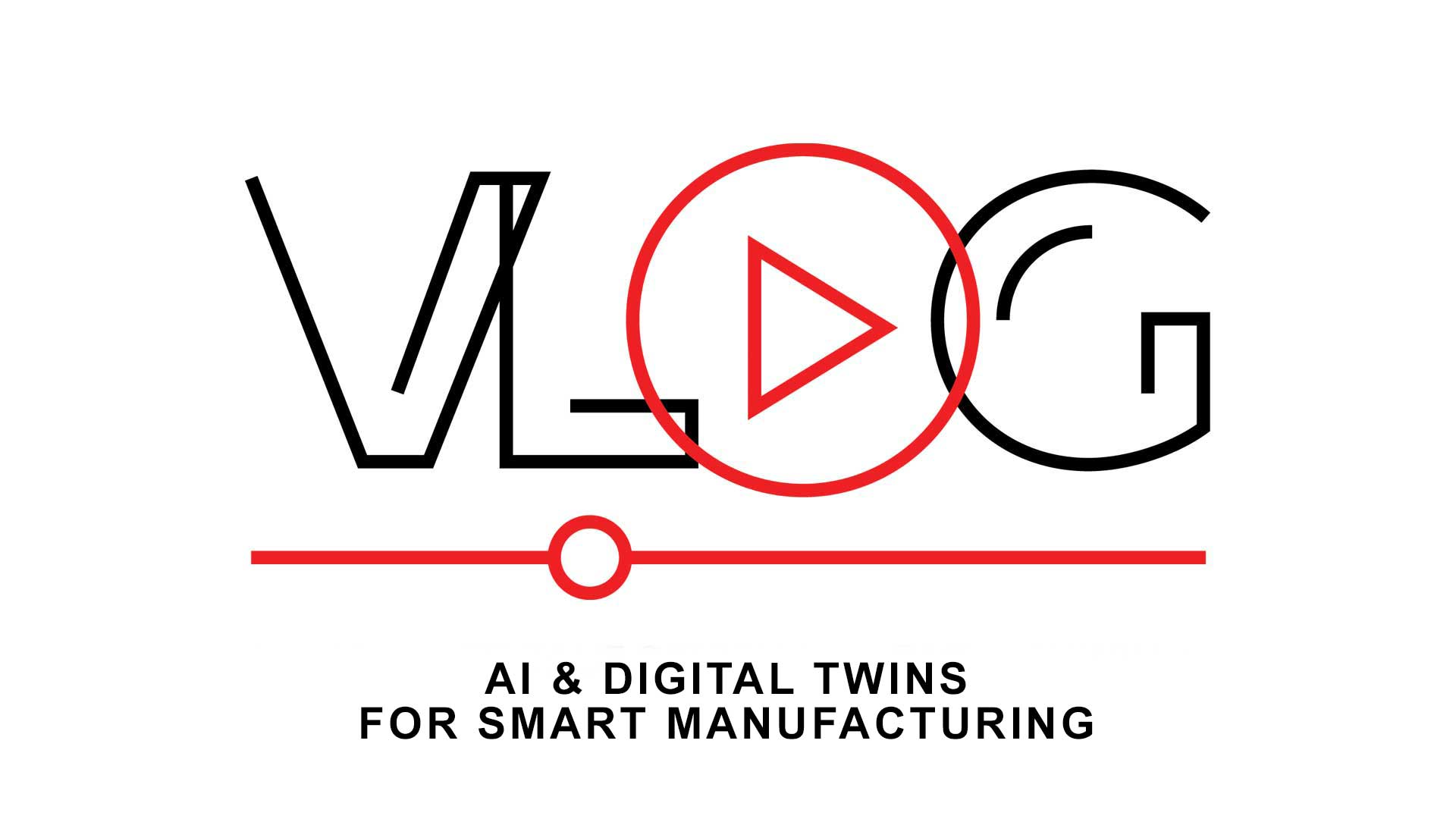
How can digital twins and artificial intelligence (AI) be used to develop production systems? Let’s talk about that next. Hey there, and welcome. If you’re looking for guidance on technology-led initiatives, you might want to think about subscribing below to our channel, or to our company page, to get insights like this on a frequent basis.
Today we will talk about how you can use digital twins and artificial intelligence to help with the development of production systems that you see in plants and facilities. Obviously, there is a big movement with smart manufacturing to stream data from equipment on the shop floor; trying to improve product quality, trying to improve throughput, and trying to shorten the time to commission production systems. We’ll talk about how all that intersects today.
To get started, let’s look at a little graphic. That will give us some background on what exactly we’re talking about. So, we usually have an existing production system that you will stream data off of. Now, you could do this with existing equipment, and you could use this with new equipment. The idea is that you capture data, and there are many hidden insights in this data to address the issues we’ve talked about before.
Now, the digital twin aspect of this comes into play when you feed that data into these 1-D analyses. These are equations or models that mimic the behavior of the equipment that you have on the shop floor, in terms of throughput. Maybe it has to deal with issues that might come up with the equipment. It could even look at the wear on that equipment. And that is your digital twin. So you have this digital, or virtual representation of your equipment that’s on a shop floor that you’re feeding sensor data as an input. That’s a key component.
So let’s take a break there for a second and kinda talk through this type of concept. This digital twin is being used in lots of different places, but in general, you have a digital representation that’s mimicking or predicting the behavior of a physical thing. Kind of its equivalent. You can do this for products, you can do this for equipment and production systems. You can do this for supply chains, and you can even do it for human bodies. There are many different examples. But here we’re talking about production systems.
Now, let’s talk about the implications, and why you would want to do that. Once you have this simulation running, that is a digital twin of your production system, you can then instrument that to get more data. Not only do you get the stream of physical sensor data that is coming off of the equipment in the real world, but you also stream virtual sensor data off of the simulation to augment that stream of real-world sensor data. You can capture this in a database, and this will turn into big data because there’s a lot of it! The idea is that you can then perform analytics. You look at the data, you try to identify where the anomalies might be, and that will let you do all sorts of interesting things around product quality, equipment uptime and things like that.
So, let’s talk a little more about this. This is a great idea in concept. A challenge is that this is a lot of data. One issue is getting analytics tools to actually handle that much data. And, you know, that’s a very tactical type of issue. A more strategic issue is, how do you find the anomalies? Buried within this sea of data are hints and clues that something’s not quite going right. Or there’s an issue with throughput, or there is going to be an issue with product quality. The question is, how do you find it? How do you find the needle in the haystack? That is the big challenge. And this is where AI comes into play and can lend a hand.
The idea here is that you release AI on this data and start looking for anomalies. You also would track events that fall kind of outside of this data, right? If there’s an issue with product quality, or if you have a regulatory compliance issue, or if you have a throughput issue, it lets the AI know, “hey, this event happened”. It then tries to correlate anomalies in the data to those events. In some cases, you’ll have situations where there are hints and clues in both the real world data and the digital twin data. The virtual sensor data, like, on the top. In some cases, there will only be clues in the real-world data, and you can identify those. The reason you want a digital twin is the case on the bottom, where the hint, or clue, only existed in the digital twin data. It’s coming off of a virtual sensor and there was no hint that this was going to happen beforehand.
So this is where you see the intersection of AI – in dealing with all of this data, finding the anomalies and doing a correlation to events with the digital twin, the simulation that’s augmenting the sensor data that you’re already capturing. In between the two of them, you get a more complete picture. You can find those issues faster and take corrective action. That’s where the intersection of artificial intelligence and the digital twin can help realize the value of connected smart manufacturing strategies more quickly. That’s it. Take care, and talk soon.